Program
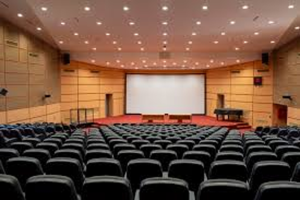
The summer school will dive into key tools in econometrics and causal analysis, starting with modern methods of instrumental variable regression to tackle issues of endogeneity in economic data. We’ll cover difference-in-differences methods, including recent advancements that handle more complex treatment scenarios. Synthetic controls will be explored as a go-to approach for case study comparisons, ideal for evaluating policy impacts. Finally, sessions on machine learning in econometrics will offer practical ways to manage large datasets and improve predictive accuracy, blending traditional techniques with modern data insights
Topics covered
• Instrumental Variable Regressions
• Difference in Difference (DiD)
• Synthetic Control Method
• Machine Learning
• Instrumental Variable Regressions
• Difference in Difference (DiD)
• Synthetic Control Method
• Machine Learning
The Summer School consists of both theoretical sessions (in the form of lectures) and practical sessions. The practical sessions take place in a computer lab. The goal is to familiarize participants with the theory and application of modern econometric evaluation techniques, including guided lab sessions. The working language is English.
Participants will have the opportunity to replicate published studies, learn the methods, and apply econometric techniques in STATA to conduct their own research. In this way, they will acquire modern S.T.A.T.A. background knowledge and apply it to their respective fields of interest.
Participants will have the opportunity to replicate published studies, learn the methods, and apply econometric techniques in STATA to conduct their own research. In this way, they will acquire modern S.T.A.T.A. background knowledge and apply it to their respective fields of interest.
Course Outline - Program
Sunday, June 22, 2025
Arrival of the participants, Registration and Welcome Reception at 19:00 at Anargyrios and Korgialenios School of Spetses Foundation – Spetses
(https://akss.gr/main/en/ )
Description: This course covers causal inference for cross-section and panel data using the potential outcomes framework. We will cover a variety of setups, including unconfoundedness (selection on observables), local treatment effects (endogeneity), and difference-in-differences. We will also cover a variety of estimation strategies, including regression adjustments, inverse probability weighting, and double robust methods. These discussions allow us to understand how to leverage advances in machine learning to conduct causal inference. A common and important theme of the course is that we will embrace treatment effect heterogeneity from the beginning and discuss estimation strategies targeting ex-ante and well-defined causal parameters. Thus, by design, we will avoid some challenges associated with reverse-engineering a plausible causal interpretation of misspecified regression coefficients. Nonetheless, we will explain how some of these pitfalls appear (and how to avoid them by design).
We will start the course by covering the identification of key treatment effects parameters assuming unconfoundedness, and then study various estimators: regression adjustment, inverse probability weighting, combinations thereof (to achieve double robustness), and matching methods. More recent methods using covariate balancing to obtain the propensity score will also be covered. We will then study the identification of the local average treatment effect (LATE) and the local average treatment effect on the treated (LATT) parameters and cover recent doubly robust methods for estimating these parameters when one has covariates in the instrument propensity score. Control function methods can be used to allow heterogeneity when functional forms are imposed on the conditional mean functions.
Once the foundation behind these different estimating strategies is solidified, we will explore how recent machine learning advances can help us more flexibly estimate treatment effect parameters under the unconfoundedness and local treatment effect paradigms. Heuristically, we will discuss the importance of using a double robust formulation of the problem to avoid biases that can affect the reliability of the estimators and their inference procedures. We will then illustrate how one can use different ML-based estimators such as LASSO, Random Forest, and Boosting to estimate ATE and LATE. We will briefly discuss strategies for flexibly estimating heterogeneous treatment effects.
We then turn to difference-in-differences methods for panel data, starting with a review of the two-period analysis. We will cover regression-based methods, propensity score methods, and doubly robust estimators for the average treatment effect on the treated (ATT). With common timing but multiple control and treatment periods, different effects can be estimated using regression methods or combining regression with inverse probability weighting. We will also discuss how to test and adjust for potential violations of a parallel trends assumption.
For the general staggered intervention case, we will discuss how to allow full heterogeneity in treatment effects by treatment cohort and calendar time. Various estimators turn out to be equivalent, including an extended version of two-way fixed effects. We will show that covariates can be easily incorporated in a regression setting or using propensity scores and double robust procedures. We will also discuss the relationship between some staggered DiD estimators and how to produce event study estimates and plots.
Finally, we will discuss some caveats related to traditional event-study-based tests for the plausibility of a parallel trends assumption and discuss how one can conduct sensitivity analysis involving relaxations of parallel trends. We will also briefly discuss alternative methods to DiD that can be used when parallel trends is not a plausible assumption.
Participants should have a good working knowledge of ordinary least squares estimation, fixed effects estimation, and basic nonlinear models such as logit, probit, and exponential conditional means. Sufficient background is provided in J.M. Wooldridge, Introductory Econometrics: A Modern Approach, 7e, Cengage, 2020.
Monday, June 23, 2025
8:30 – 9:30 Registration09:30-11:00 Lecture 1 (Jeffrey M. Wooldridge)
11:00-11:30 Coffee break
11:30-13:00 Lecture 2 (Pedro Sant’Anna)
13:00 -14:30 Lunch
14:30—16:00 Lab Session 1
Tuesday, June 24, 2025
09:30-11:00 Lecture 3 (Jeffrey M. Wooldridge)11:00-11:30 Coffee break
11:30-13:00 Lecture 4 (Pedro Sant’Anna)
13:00 -14:30 Lunch
14:30—16:00 Lab Session 2
Wednesday, June 25, 2025
09:30-11:00 Lecture 5 (Jeffrey M. Wooldridge)11:00-11:30 Coffee break
11:30-13:00 Lecture 6 (Pedro Sant’Anna)
13:00 -14:30 Lunch
14:30—16:00 Lab Session 3
Thursday, June 26, 2025
09:30-11:00 Lecture 7 (Jeffrey M. Wooldridge)11:00-11:30 Coffee break
11:30-13:00 Lecture 7 (Pedro Sant’Anna)
13:00 -14:30 Lunch
14:30—16:00 Lab Session 4
20:00 Farewell dinner
Friday, June 27, 2025
09:30-11:00 Lecture 9 (Jeffrey M. Wooldridge)11:00-11:30 Coffee break
11:30-13:00 Lecture 10 (Pedro Sant’Anna)
13:00 -14:30 Lunch
14:30—16:00 Lab Session 5
16:00 – 17:00 Optional Poster Presentation of Participants (PhD candidates and young researchers)
Daily Content
Potential outcomes and parameters of interest. Randomization. Unconfoundedness and overlap. Identification. Regression adjustment, inverse probability weighting, normalized weights. Covariate balancing propensity score estimation. Matching and doubly robust estimators. Improved efficiency under randomized controlled trials. Multiple treatments.
Day 2
Potential treatment status. Constant effect model. Compliers and defiers. One-sided non-compliance. Identification of LATE and LATT. Including covariates for LATE and LATT estimation. Control function methods.
Day 3
Introduction to Causal Machine Learning. Importance of using double robust procedures. LASSO, Random Forest, Boosting, and other ML estimators. Cross-fitting. Heterogenous Treatment Effects Estimation.
Two-period difference-in-differences; no anticipation and parallel trends; controlling for covariates via regression adjustment and propensity score methods. General common timing case and heterogeneous effects. Functional Form.
Day 4
Staggered interventions. Parameters of interest. Parallel trends. Shortcomings of the constant effect model. Regression-based methods. Imputation. Callaway and Sant’Anna long-difference approach and doubly robust estimators. Efficient gains by exploring more pre-treatment information.
Day 5
Event Study Methods and Testing for Pre-Trends. Estimation with Heterogeneous Trends. Sensitivity analysis for violation of parallel trends. Unconfoundedness given pre-treatment outcomes. Synthetic Control and Synthetic Difference-in-Differences.
Reading List
1General Background Reading
de Chaisemartin, C. and X. D’Haultfœuille (2023), “Two-way fixed effects and differences-in-differences with heterogeneous treatment effects: a survey,” Econometrics Journal 26, C1–C30.
Imbens, G.W. and J.M. Wooldridge (2009), “Recent Developments in the Econometrics of Program Evaluation,” Journal of Economic Literature. (IW2009)
Roth, J., P.H.C. Sant’Anna, A. Bilinski, and J. Poe (2023), “What's Trending in Difference-in-Differences? A Synthesis of the Recent Econometrics Literature,” Journal of Econometrics 235, 2218-2244.
Imbens, G.W. and J.M. Wooldridge (2009), “Recent Developments in the Econometrics of Program Evaluation,” Journal of Economic Literature. (IW2009)
Roth, J., P.H.C. Sant’Anna, A. Bilinski, and J. Poe (2023), “What's Trending in Difference-in-Differences? A Synthesis of the Recent Econometrics Literature,” Journal of Econometrics 235, 2218-2244.
2Day 1
Angrist, J.D. and J.-S. Pischke (2009), Mostly Harmless Econometrics. Princeton University Press. Chapters 1 through 3.
Cunningham, S. (2021), Causal Inference: The Mixtape. Yale University Press. Chapter 4.
Graham, B., C. Pinto, and D. Egel (2012), “Inverse Probability Tilting for Moment Condition Models with Missing Data,” The Review of Economic Studies 79, 1053-1079.
Graham, B., C. Pinto, and D. Egel (2016), “Efficient Estimation of Data Combination Models by the Method of Auxiliary-to-Study Tilting (AST),” Journal of Business & Economic Statistics 34, 288-301.
Hahn, J. (1998), “On the Role of the Propensity Score in Efficient Semiparametric Estimation of Average Treatment Effects”, Econometrica 66, 315-331.
Imai, K. and M. Ratkovic (2014), “Covariate Balancing Propensity Score,” Journal of the Royal Statistical Society: Series B 76, 243-263.
Imbens, G.W. and D.B. Rubin (2015), Causal Inference for Statistics, Social, and Behavioral Sciences. Cambridge University Press. Chapters 1-7, 12-13.
Negi, A. and J.M. Wooldridge (2021), “Revisiting Regression Adjustment in Experiments with Heterogeneous Treatment Effects,” Econometric Reviews 40, 504-534.
Słoczyński, T. (2022), “Interpreting Estimands when Treatment Effects are Heterogeneous: Smaller Groups Get Larger Weights,” Review of Economics and Statistics 104, 501-509.
Słoczyński, T., S.D. Uysal, and J.M. Wooldridge (2023), “Covariate Balancing and the Equivalence of Weighting and Doubly Robust Estimators of Average Treatment Effects,” working paper. https://arxiv.org/pdf/2310.18563
Wooldridge, J.M. (2010), Econometric Analysis of Cross Section and Panel Data, MIT Press. Chapter 21.
Cunningham, S. (2021), Causal Inference: The Mixtape. Yale University Press. Chapter 4.
Graham, B., C. Pinto, and D. Egel (2012), “Inverse Probability Tilting for Moment Condition Models with Missing Data,” The Review of Economic Studies 79, 1053-1079.
Graham, B., C. Pinto, and D. Egel (2016), “Efficient Estimation of Data Combination Models by the Method of Auxiliary-to-Study Tilting (AST),” Journal of Business & Economic Statistics 34, 288-301.
Hahn, J. (1998), “On the Role of the Propensity Score in Efficient Semiparametric Estimation of Average Treatment Effects”, Econometrica 66, 315-331.
Imai, K. and M. Ratkovic (2014), “Covariate Balancing Propensity Score,” Journal of the Royal Statistical Society: Series B 76, 243-263.
Imbens, G.W. and D.B. Rubin (2015), Causal Inference for Statistics, Social, and Behavioral Sciences. Cambridge University Press. Chapters 1-7, 12-13.
Negi, A. and J.M. Wooldridge (2021), “Revisiting Regression Adjustment in Experiments with Heterogeneous Treatment Effects,” Econometric Reviews 40, 504-534.
Słoczyński, T. (2022), “Interpreting Estimands when Treatment Effects are Heterogeneous: Smaller Groups Get Larger Weights,” Review of Economics and Statistics 104, 501-509.
Słoczyński, T., S.D. Uysal, and J.M. Wooldridge (2023), “Covariate Balancing and the Equivalence of Weighting and Doubly Robust Estimators of Average Treatment Effects,” working paper. https://arxiv.org/pdf/2310.18563
Wooldridge, J.M. (2010), Econometric Analysis of Cross Section and Panel Data, MIT Press. Chapter 21.
3Day 2
Abadie, A. (2003), “Semiparametric Instrumental Variable Estimation of Treatment
Response Models,” Journal of Econometrics 113 231-263.
Angrist and Pischke (2009), Chapter 4.
Cunningham, S. (2021), Causal Inference: The Mixtape. Yale University Press. Chapter 7.
Imbens, G.W. and J.D. Angrist (1994), “Identification and Estimation of Local Average Treatment Effects,” Econometrica 62, 467-475.
Imbens and Rubin (2015), Chapters 23-24.
Frölich, M. (2007), “Nonparametric IV Estimation of Local Average Treatment Effects with Covariates,” Journal of Econometrics 139, 35-75.
Heiler, P. (2022), “Efficient Covariate Balancing for the Local Average Treatment Effect,” Journal of Business & Economic Statistics 40, 1569-1582.
Sant’Anna, P. H. C., X. Song, and Q. Xu (2022), “Covariate Distribution Balance via Propensity Scores,” Journal of Applied Econometrics 37, 1093-1120.
Słoczyński, T., S.D. Uysal, and J.M. Wooldridge (2022), “Doubly Robust Estimation of Local Average Treatment Effects Using Inverse Probability Weighted Regression Adjustment.”
Angrist and Pischke (2009), Chapter 4.
Cunningham, S. (2021), Causal Inference: The Mixtape. Yale University Press. Chapter 7.
Imbens, G.W. and J.D. Angrist (1994), “Identification and Estimation of Local Average Treatment Effects,” Econometrica 62, 467-475.
Imbens and Rubin (2015), Chapters 23-24.
Frölich, M. (2007), “Nonparametric IV Estimation of Local Average Treatment Effects with Covariates,” Journal of Econometrics 139, 35-75.
Heiler, P. (2022), “Efficient Covariate Balancing for the Local Average Treatment Effect,” Journal of Business & Economic Statistics 40, 1569-1582.
Sant’Anna, P. H. C., X. Song, and Q. Xu (2022), “Covariate Distribution Balance via Propensity Scores,” Journal of Applied Econometrics 37, 1093-1120.
Słoczyński, T., S.D. Uysal, and J.M. Wooldridge (2022), “Doubly Robust Estimation of Local Average Treatment Effects Using Inverse Probability Weighted Regression Adjustment.”
4Day 3
Abadie, A. (2005), “Semiparametric Difference-in-Differences Estimators,” Review of Economic Studies 72, 1-19.
Ahrens, A., C. Hansen, M. E. Schaffer and T. Wiemann (2024), “Model Averaging and Double Machine Learning”, arXiv:2401.01645
Angrist and Pischke (2009), Chapter 5.
Belloni, A., V. Chernozhukov, and C. Hansen, (2014), “Inference on Treatment Effects after Selection among High-Dimensional Controls”, The Review of Economic Studies 85, 233-298.
Belloni, A., V. Chernozhukov, I. Fernandez-Val, and C. Hansen, (2017), “Program Evaluation and Causal Inference with High-Dimensional Data”, Econometrica 81, 608-650.
Chernozhukov, V., D. Chetverikov, M. Demirer, E. Duflo, C. Hansen, W. Newey, Whitney and J. Robins (2018), “Double/Debiased Machine Learning for Treatment and Structural Parameters” Econometrics Journal 21, C1-C68.
Cunningham, S. (2021), Causal Inference: The Mixtape. Yale University Press. Chapter 9.
Farrell, M. H. (2015), “Robust Inference on Average Treatment Effects with Possibly More Covariates than Observations,” Journal of Econometrics 189, 1–23.
Heckman, J.J., H. Ichimura, and P.E. Todd (1997), “Matching as an Econometric Evaluation Estimator: Evidence from Evaluating a Job Training Programme,” Review of Economic Studies 64, 605-654.
Lee, S.J., and J.M. Wooldridge (2023), “A Simple Transformation Approach to Difference-in-Differences Estimation for Panel Data,” working paper. https://papers.ssrn.com/sol3/papers.cfm?abstract_id=4516518
Roth, J. and Sant’Anna (2023), “When is Parallel Trends Sensitive to Functional Form?” Econometrica 2, 737-747.
Sant’Anna, P.H.C. and J. Zhao (2020), “Doubly Robust Difference-in-Differences Estimators,” Journal of Econometrics 219, 101-122.
Semenova, V. and V. Chernozhukov (2021), “Debiased Machine Learning of Conditional Average Treatment Effects and Other Causal Functions,” Econometrics Journal 24, 264 – 289
Tan, Z. (2020), “Model-Assisted Inference for Treatment Effects Using Regularized Calibrated Estimation with High-Dimensional Data”, Annals of Statistics 48, 811-837.
Wager, S. and S. Athey (2018), “Estimation and Inference of Heterogeneous Treatment Effects using Random Forests”, Journal of the American Statistical Association 113, 1228 – 1242.
Ahrens, A., C. Hansen, M. E. Schaffer and T. Wiemann (2024), “Model Averaging and Double Machine Learning”, arXiv:2401.01645
Angrist and Pischke (2009), Chapter 5.
Belloni, A., V. Chernozhukov, and C. Hansen, (2014), “Inference on Treatment Effects after Selection among High-Dimensional Controls”, The Review of Economic Studies 85, 233-298.
Belloni, A., V. Chernozhukov, I. Fernandez-Val, and C. Hansen, (2017), “Program Evaluation and Causal Inference with High-Dimensional Data”, Econometrica 81, 608-650.
Chernozhukov, V., D. Chetverikov, M. Demirer, E. Duflo, C. Hansen, W. Newey, Whitney and J. Robins (2018), “Double/Debiased Machine Learning for Treatment and Structural Parameters” Econometrics Journal 21, C1-C68.
Cunningham, S. (2021), Causal Inference: The Mixtape. Yale University Press. Chapter 9.
Farrell, M. H. (2015), “Robust Inference on Average Treatment Effects with Possibly More Covariates than Observations,” Journal of Econometrics 189, 1–23.
Heckman, J.J., H. Ichimura, and P.E. Todd (1997), “Matching as an Econometric Evaluation Estimator: Evidence from Evaluating a Job Training Programme,” Review of Economic Studies 64, 605-654.
Lee, S.J., and J.M. Wooldridge (2023), “A Simple Transformation Approach to Difference-in-Differences Estimation for Panel Data,” working paper. https://papers.ssrn.com/sol3/papers.cfm?abstract_id=4516518
Roth, J. and Sant’Anna (2023), “When is Parallel Trends Sensitive to Functional Form?” Econometrica 2, 737-747.
Sant’Anna, P.H.C. and J. Zhao (2020), “Doubly Robust Difference-in-Differences Estimators,” Journal of Econometrics 219, 101-122.
Semenova, V. and V. Chernozhukov (2021), “Debiased Machine Learning of Conditional Average Treatment Effects and Other Causal Functions,” Econometrics Journal 24, 264 – 289
Tan, Z. (2020), “Model-Assisted Inference for Treatment Effects Using Regularized Calibrated Estimation with High-Dimensional Data”, Annals of Statistics 48, 811-837.
Wager, S. and S. Athey (2018), “Estimation and Inference of Heterogeneous Treatment Effects using Random Forests”, Journal of the American Statistical Association 113, 1228 – 1242.
5Day 4
Borusyak, K., X. Jaravel, and J. Spiess (2024), “Revisiting Event Study Designs: Robust and Efficient Estimation,” Review of Economic Studies 91, 3253-3285.
Callaway, B., and P.H.C. Sant’Anna (2021), “Difference-in-Differences with Multiple Time Periods,” Journal of Econometrics 225, 200-230.
de Chaisemartin, C., and X. D'Haultfœuille (2020), “Two-Way Fixed Effects Estimators with Heterogeneous Treatment Effects,” American Economic Review 110, 2964-2996.
Goodman-Bacon, A. (2021), “Difference-in-Differences with Variation in Treatment Timing,” Journal of Econometrics 225, 254-277.
Sun, L., and S. Abraham (2021), “Estimating Dynamic Treatment Effects in Event Studies with Heterogeneous Treatment Effects,” Journal of Econometrics 202, 175-199
Wooldridge, J.M. (2024), “Two-Way Fixed Effects, the Two-Way Mundlak Regression, and Difference-in-Differences Estimators,” working paper. https://www.dropbox.com/scl/fi/u4wua01rmsyzcqee343gy/two_way_mundlak_20241229.pdf?rlkey=nxrangwq0eww1hfdo2hs22vfi&dl=0
Callaway, B., and P.H.C. Sant’Anna (2021), “Difference-in-Differences with Multiple Time Periods,” Journal of Econometrics 225, 200-230.
de Chaisemartin, C., and X. D'Haultfœuille (2020), “Two-Way Fixed Effects Estimators with Heterogeneous Treatment Effects,” American Economic Review 110, 2964-2996.
Goodman-Bacon, A. (2021), “Difference-in-Differences with Variation in Treatment Timing,” Journal of Econometrics 225, 254-277.
Sun, L., and S. Abraham (2021), “Estimating Dynamic Treatment Effects in Event Studies with Heterogeneous Treatment Effects,” Journal of Econometrics 202, 175-199
Wooldridge, J.M. (2024), “Two-Way Fixed Effects, the Two-Way Mundlak Regression, and Difference-in-Differences Estimators,” working paper. https://www.dropbox.com/scl/fi/u4wua01rmsyzcqee343gy/two_way_mundlak_20241229.pdf?rlkey=nxrangwq0eww1hfdo2hs22vfi&dl=0
6Day 5
Abadie, A., A. Diamond, and J. Hainmueller (2010), “Synthetic control methods for comparative case studies: Estimating the effect of California’s tobacco control program”. Journal of the American Statistical Association 105, 493–505.
Arkhangelsky, D., S. Athey, D. A. Hirshberg, G. W. Imbens, and S. Wager (2021), “Synthetic difference-in-differences,” American Economic Review 111, 4088–4118.
Athey, S., M. Bayati, N. Doudchenko, G. Imbens, and K. Khosravi (2021), “Matrix completion methods for causal panel data models,” Journal of the American Statistical Association 116, 1716-1730.
Ben-Michael, E., A. Feller, and J. Rothstein (2021), “The Augmented Synthetic Method”, Journal of the American Statistical Association 116, 1789-1803
Ben-Michael, E., A. Feller, and J. Rothstein (2022), “Synthetic Controls with Staggered Adoption”, Journal of the Royal Statistical Society: Series B 84, 351-381
Caetano, C., and B. Callaway (2024), “Difference-in-Differences with Time-Varying Covariates in the Parallel Trends Assumption,” arXiv:2406.15288
Callaway, B., and P.H.C. Sant’Anna (2021), “Difference-in-Differences with Multiple Time Periods,” Journal of Econometrics 225, 200-230.
Ding, P., and F. Li (2019), “A Bracketing Relationship between Difference-in-Differences and Lagged-Dependent-Variable Adjustment,” Political Analysis 27, 605-615.
Freyaldenhoven, S., C. Hansen, and J. M. Shapiro (2019), “Pre-event trends in the panel event-study design,” American Economic Review 109, 3307–38.
Gobillon, L. and T. Magnac (2015), “Regional Policy Evaluation: Interactive Fixed Effects and Synthetic Controls,” The Review of Economics and Statistics 98, 535-551.
Roth, J. (2022), ‘Pretest with Caution: Event-Study Estimates after Testing for Parallel Trends,” American Economic Review: Insights 4, 305-322.
Rambachan, A., and J. Roth (2023), “A More Credible Approach to Parallel Trends,” Review of Economic Studies 90, 2555–2591.
Sant’Anna, P. H. C., and Q. Xu (2024), “Difference-in-Differences with Compositional Changes”, arXiv:2304.14256.
Viviano, D. and J. Bradic (2024), “Dynamic covariate balancing: estimating treatment effects over time,” arXiv:2103.01280
Wooldridge, J.M. (2024), “Two-Way Fixed Effects, the Two-Way Mundlak Regression, and Difference-in-Differences Estimators,” working paper. https://www.dropbox.com/scl/fi/u4wua01rmsyzcqee343gy/two_way_mundlak_20241229.pdf?rlkey=nxrangwq0eww1hfdo2hs22vfi&dl=0
Wooldridge, J. M. (2005), “Fixed-effects and related estimators for correlated random-coefficient and treatment-effect panel data models,” Review of Economics and Statistics 87, 385–390.
Xu, Y. (2017), “Generalized synthetic control method: Causal inference with interactive fixed effects models,” Political Analysis 25, 57–76.
Arkhangelsky, D., S. Athey, D. A. Hirshberg, G. W. Imbens, and S. Wager (2021), “Synthetic difference-in-differences,” American Economic Review 111, 4088–4118.
Athey, S., M. Bayati, N. Doudchenko, G. Imbens, and K. Khosravi (2021), “Matrix completion methods for causal panel data models,” Journal of the American Statistical Association 116, 1716-1730.
Ben-Michael, E., A. Feller, and J. Rothstein (2021), “The Augmented Synthetic Method”, Journal of the American Statistical Association 116, 1789-1803
Ben-Michael, E., A. Feller, and J. Rothstein (2022), “Synthetic Controls with Staggered Adoption”, Journal of the Royal Statistical Society: Series B 84, 351-381
Caetano, C., and B. Callaway (2024), “Difference-in-Differences with Time-Varying Covariates in the Parallel Trends Assumption,” arXiv:2406.15288
Callaway, B., and P.H.C. Sant’Anna (2021), “Difference-in-Differences with Multiple Time Periods,” Journal of Econometrics 225, 200-230.
Ding, P., and F. Li (2019), “A Bracketing Relationship between Difference-in-Differences and Lagged-Dependent-Variable Adjustment,” Political Analysis 27, 605-615.
Freyaldenhoven, S., C. Hansen, and J. M. Shapiro (2019), “Pre-event trends in the panel event-study design,” American Economic Review 109, 3307–38.
Gobillon, L. and T. Magnac (2015), “Regional Policy Evaluation: Interactive Fixed Effects and Synthetic Controls,” The Review of Economics and Statistics 98, 535-551.
Roth, J. (2022), ‘Pretest with Caution: Event-Study Estimates after Testing for Parallel Trends,” American Economic Review: Insights 4, 305-322.
Rambachan, A., and J. Roth (2023), “A More Credible Approach to Parallel Trends,” Review of Economic Studies 90, 2555–2591.
Sant’Anna, P. H. C., and Q. Xu (2024), “Difference-in-Differences with Compositional Changes”, arXiv:2304.14256.
Viviano, D. and J. Bradic (2024), “Dynamic covariate balancing: estimating treatment effects over time,” arXiv:2103.01280
Wooldridge, J.M. (2024), “Two-Way Fixed Effects, the Two-Way Mundlak Regression, and Difference-in-Differences Estimators,” working paper. https://www.dropbox.com/scl/fi/u4wua01rmsyzcqee343gy/two_way_mundlak_20241229.pdf?rlkey=nxrangwq0eww1hfdo2hs22vfi&dl=0
Wooldridge, J. M. (2005), “Fixed-effects and related estimators for correlated random-coefficient and treatment-effect panel data models,” Review of Economics and Statistics 87, 385–390.
Xu, Y. (2017), “Generalized synthetic control method: Causal inference with interactive fixed effects models,” Political Analysis 25, 57–76.
